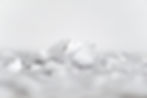
Epistemic Uncertainty and Data-Driven Policy Making
Policy driven by data and science represents the gold standard amongst politicians of all political stripes who seek to break through the partisan gridlock. From health and safety protocols surrounding COVID-19 to long term projects related to infrastructure and public transit, the ideal appears to be to let the data “speak for itself” and lead the way. In this post, we examine the relationship between science, scientific practices and policy making, and highlight one aspect of this relationship that has been underappreciated: namely, the relationship between science and policy making is symbiotic not unidirectional. Implicit and explicit value assumptions made by policymakers and scientists alike inevitably frame and shape the data we collect. As a result, we argue the relevant roles of scientists and policymakers have often been misunderstood, and appreciating the symbiotic relationship that exists between these two sets of actors may indicate a need to reform policymaking institutions such as the FDA and CDC.
The Promise of Objectivity and the Value-Free Ideal
To begin, advocates tend to promote two advantages of data-driven policymaking. The first advantage is that it appears to be an attractive response to a political environment that increasingly breaks down along partisan and ideological divides. As MITRE notes on their website, “MITRE’s Center for Data-Driven Policy brings objective, evidence-based, nonpartisan insights to government policymaking.” MITRE, and organizations like it, then, see data-driven policy as a way out of these ideological debates: allowing politicians (and the public that supports them) to work together and make progress toward legislation that promotes a set of shared interests (e.g., a strong economy).
Another advantage of data-driven policymaking is that it appears to be particularly well-suited to responding to the problem of scarcity. Given governments have limited money to spend, politicians have a moral obligation to put forward policies and proposals that can generate the best-return on investment: whether that be traditional infrastructure or other public investments (e.g., education). The promise of data-driven policymaking, therefore, is that it can allow us to effectively calculate how best to spend these limited resources. As Lauren Greenawalt quips in her 2018 article, “[a]s the thinking goes, you can’t manage what you can’t measure.” And when policymakers don’t have access to the relevant data to evaluate existing projects or develop new ones, “[e]ither way, the public loses: Policymakers miss an opportunity to advance good policy, taxpayers don’t see a return on their investment, and those whom a policy is intended to help aren’t served.”
The problem, of course, is that both advantages assume data to be objective; and that policymaking which ‘follows the data’ is simply responding to the evidence about what the world ‘out there’ is really like. Unfortunately, despite the prevalence of the folk view that data and science are value-free, objective enterprises, philosophers of science have long argued that values are integral to the practice of science and data-gathering. For example, Helen Longino (1996) has argued that the so-called “cognitive” values are not always politically neutral. For instance, appeals to simplicity when deciding between two competing theories (e.g., explanations of factors that contribute to student success) is not a politically neutral criteria, but privileges a certain set of values over others (e.g., efficiency over equity) and can risk overgeneralization at the level of policy making (e.g., the darling of William MacAskill’s (2015) effective altruism Deworm The World). Similarly, John Dupré (2007) has argued that because scientific theories emerge from human culture the language of our theories will inevitably contain “thick” concepts (e.g., ‘species’). As a result, our theories of the world (e.g., evolution) do not simply describe the world ‘as it is,’ but necessarily take on many implicit and explicit assumptions baked into our cultures and languages.
As a result, despite the promise that evidenced-based policy making can lead us out of the messy world of values, data, science, and scientific practices are value-laden—even prior being used as inputs for crafting policy in governments and organizations. Of course, it does not follow that data and evidence shouldn’t play any role in informing the decisions of policymakers; rather, we seek to highlight the way in which values play an inextricable role in these decisions and processes.
The Roles of Scientists and Policymakers
The argument above indicates that at least one role practicing scientists ought to be cognizant of is the role their work plays in the policymaking process. For example, Douglas (2000) rightly notes that because the possibility of error for a given scientific finding is never null (inductive risk), scientists ought to be responsive to non-epistemic risks of error. Non-epistemic risks are those consequences of error that extend beyond “being wrong”, and include material harms. For example, when building a pedestrian bridge the inductive risk associated with whether it will collapse isn’t merely an epistemic risk for the engineer, but a moral one. Indeed, one reason engineers (or scientists in general) must be cognizant of inductive risks is because these risks can have grave consequences at the level of policy and policymaking—i.e., people can die.
However, this overlooks an important part of the relationship between data, science, and policymaking: namely, the relationship is symbiotic. We believe many philosophers of science implicitly endorse this view. That said, when we emphasize the epistemic and moral responsibilities of scientists to be sensitive to inductive risks, we believe this can inadvertently mask the ways in which policymakers play an active role in the practices of science as well. For example, since 2003 Tiahrt Amendments to the US Department of Justice appropriations bills have significantly restricted the Bureau of Alcohol, Tobacco, Firearms and Explosives to collect and release data pertaining to gun dealers and gun crimes. In this way, policymaking isn’t exclusively an output (or potential output) of data collection and scientific practices, but policy and policymakers are playing an active role in shaping the kind of data available to be collected in the first place.
Of course, the Tiahrt Amendments have garnered much attention, and, in recent years, congress has arguably weakened some of these prohibitions. Nevertheless, it is important to note that policymakers playing this kind of active role in the practices of science is not an isolated incident. For example, Cailin O’Connor and James Weatherall (2019) note the ways in which large government grants can create feedback loops (e.g., through placing students who then replicate their methodologies) which can lead to the widespread adoption of beliefs in worse theories. The implication here isn’t that governments and policymakers shouldn’t make such grants available; rather, it's to (once again) highlight the active role policymakers are playing in the process of science and data collection itself.
What To Do About It Now?
One relatively uncontroversial conclusion, therefore, is that, like scientists, policymakers should be responsive to the non-epistemic consequences posed by inductive risk latent in all scientific findings. In cases such as Deworm the World, it would follow that policy makers have an obligation to resist overgeneralizing from some evidence that deworming medication helps to improve test scores in children in the developing world, to policies which privileged deworming programs over and above almost all other development projects.
More controversially, we believe that policymakers should also be responsive to the epistemic consequences posed by policy decisions: particularly those where (1) the degree of inductive risk is comparatively high, and yet (2) policy makers cannot postpone decisions until the ‘results are in’ (e.g., in cases where in-action is tantamount to a decision). Indeed, we believe this responsibility follows directly from appreciating the symbiotic relationship between policymaking, data, and scientific practices. For example, in the early days of the COVID-19 pandemic there was emerging consensus in the scientific community that masks would do relatively little to curb the spread of virus. In response, policymakers across the globe broadly adopted ‘stay-at-home’ orders, and deemphasized the importance of wearing masks in public spaces. Perhaps this was the right decision; however, note that in either case an epistemic consequence of these decisions is that they contribute to the public’s trust (or distrust) in science.
In short, we believe that this epistemic relationship has gone underappreciated. In emphasizing the implications of the moral responsibilities of scientists, we have masked the ways in which policymakers play an active role in the practices of science via the way those decisions contribute to what we as a larger community “know.” The data available to us at any given time are neither a neutral nor a complete picture of the world – rather they reflect a complex combination of constraints including past policy decisions. Our largest suggestion, then, is that in thinking about how policymaking institutions like the FDA and CDC should be reshaped, we need to consider not only the ethical implications of policymaking, but the epistemic ones as well. In order to address the later of these issues, it will require these institutions not only to adopt a pluralist stance at the level of basic research, but it might be the case that at least some funding is better awarded on a lottery basis rather than ‘merit.’
References
Douglas, H. (2000). Inductive risk and values in science. Philosophy of Science, 67(4), 559–579. https://doi.org/10.1086/392855
Dupré, J. (2007). Fact and Value. In Value-free science?: Ideals and illusions (pp. 27–41). essay, Oxford University Press.
Longino, H. E. (1996). Cognitive and non-cognitive values in Science: Rethinking the dichotomy. Feminism, Science, and the Philosophy of Science, 39–58. https://doi.org/10.1007/978-94-009-1742-2_3
MacAskill, W. (2015). Doing good better: Effective altruism and a radical new way to make a difference. Guardian Faber Publishing.
O'Connor, C., & Weatherall, J. O. (2019). The misinformation age: How false beliefs spread. Yale University Press.